All simulated agricultural systems experiments attempt to mimic the behavior of real-world systems. To ensure that they represent viable systems from the perspective of practitioners, these experiments must be developed with grower participation. The research team needs to be clear during both the design and implementation phases about what systems are being represented in the experiment and should involve knowledgeable farmers in the design process. Moreover, when planning the research, keep in mind that any new management scheme will undergo a transition phase and will require time to reflect the current management practices. For example, if cover crop rotations are imposed on a site in which annual crops have been alternated with bare fallows, changes in the size and composition of the microbial community and labile soil organic matter (SOM) pools may be detected in two to four years while significant changes in total SOM pools may not be evident for a decade or more.
Defining the Systems
Once the goals and objectives are clearly established, defining the system treatments is fairly straightforward, as long as realistic management regimes are used. Realistic agricultural systems vary extensively, and comparisons of single management practices will not be possible in these experiments. While comparing practices by restricting the number of factors that differ across treatments can be tempting, this approach often results in failure because the treatments do not represent a viable agricultural system. The treatments being compared must be reasonable representations of agricultural systems. For example, to compare rotations with and without cover crops, other management practices must be modified to adjust for the addition of cover crops. Planting dates for cash crops will be later and nitrogen fertilizer rates can be reduced when cover crops replace bare fallows. Likewise, to compare realistic organic and conventional management systems, treatments must differ in many management practices such as crop rotation, inputs, crop varieties and planting dates. This may seem problematic to those who are more familiar with factorial designs in which all aspects of management except for the factor of interest are held constant. However, farmers can rarely make a single change in their farming system without adjusting other variables to optimize the system performance.
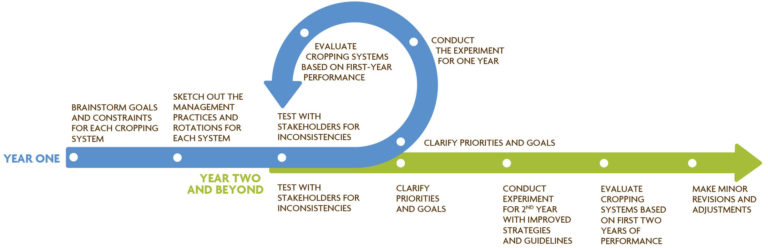
In order to develop specific management practices, the team must establish distinct objectives and guiding strategies for each agricultural system treatment. This approach can involve an iterative cycle in which scenarios are developed with farmer involvement, after which a wider group of stakeholders assesses whether the system treatments appear valid (Figure 3.3). Developing distinct guidelines for each treatment allows for flexibility in management practices to respond to normal variation through time, such as variability in precipitation or weed pressure, while ensuring that the management conforms to the overall strategy.
A good example of this process can be seen in a longterm systems experiment in New York comparing four organic vegetable cropping systems. Although all of the systems focused on organic vegetable production and included crops commonly grown in the northeastern United States, their management practices reflected differences in income goals, production constraints and overall management priorities (Table 3.2). Cropping System 1 (CS1) simulated a typical, intensive vegetable system with double cropping in two of the four years (i.e., six cash crops in a four-year cycle). CS3 mimicked an innovative, reduced-intensity system developed by two experienced organic farmers and alternated one year of cash crops with one year of cover cropping, meaning cash crops were produced in two of every four years (i.e., two cash crops in a four-year cycle). CS2 and CS4 were experimental treatments developed collaboratively by the scientists, extension educators and farmers; these treatments applied key strategies used in CS3 while aiming to produce a cash crop every year (i.e., four cash crops in a four-year cycle). The goals and constraints were similar in CS2 and CS4, but CS2 emphasized the use of cover crops, whereas CS4 involved reducing tillage.
Before developing new agricultural systems experiments, review and analyze past and ongoing systems trials established across the United States (Table 3.1); each has unique design features that reflect the regional environment, farming practices and research priorities. These experiments fall along a continuum, from those investigating fundamental questions about ecological processes to those focused on optimizing regional production systems. Virtually all of these experiments can generate both practical outcomes and new knowledge of ecological processes in agricultural systems. They have also involved farmers and include supplemental experiments in addition to the main systems experiment.
So, how does the team go about developing system treatments to be compared? As with all other design decisions, begin with the goals of the research project. If the goals are to improve or optimize production systems for particular crops, then the treatments may need to capture subtle differences. For example, the project could include organic grain treatments that differ only in terms of rotation, fertility inputs and tillage. This approach is commonly used when the most important goal is to develop improvements for a management system or to evaluate a range of options in a way that is meaningful to farmers. Box 3.2 outlines how one group developed a cropping systems experiment with these goals in mind.
Box 3.2: Using Farmer Knowledge to Explore Challenges in Potato Production Systems
In early 2001, researchers, extension staff and private crop advisers in Michigan met to address concerns among potato producers about declining yields. Farmers guessed the decreases were due to soil organic matter degradation resulting from shortened rotation sequences, minimal residue inputs and limited use of winter cover crops. Soil organic matter decline is a common problem in intensive vegetable production systems, and it is exacerbated in potato systems because growers use sandy, well-drained soils that tend to have inherently low levels of organic matter. In addition, nutrient requirements for potato production are high, often double that required by field crops. In the study, funded by the USDA Initiative for Future Agriculture and Food Systems, farmers challenged the multidisciplinary team to provide innovative approaches that could supply sufficient nutrients to crops while protecting the environment and helping operations of different sizes remain economically viable. The team included experts in horticulture, forage agronomy, soil science, plant pathology and agricultural economics. It met with an advisory group of growers, private crop consultants and a representative from the Michigan Potato Industry Commission. For nine months, the team reviewed the literature and farmer experience with alternative cropping systems including various rotational sequences, winter-cover alternatives and low-rate applications of compost to address soil declines in an economically viable manner. Initially, the team designed a long-term trial that included a factorial approach to comparing low and high fertilizer nitrogen levels applied to eight potato-rotation sequences with different intensities of residues and winter cover. Potato growers, however, were not interested in this design, because it was complex and had a large number of treatments, including many that were biologically interesting but not viable in terms of farming integrity or economics. The growers were interested in an experiment that compared intact cropping systems. As a result, the final experimental design, developed in response to input from growers and cooperative extension, was revised to compare cropping systems that included three common farmer rotations as “goal posts” to a farmer cover crop rotation that kept the soil continuously covered and produced large amounts of cover crop residues. Also included were four researcher-designed and farmer-approved alternative rotations that tested gradients of biodiversity via the presence of diverse cover crops and treatments with or without compost. Two critical elements in this process turned out to be (1) a baseline survey to document farmer practice in pilot areas at the beginning of the project, allowing evaluation of impact and adoption over time; and (2) frequent input from the farmer advisors provided through surveys, informal discussions at the trial sites and formal meetings with the research team.
Sieglinde Snapp, Project Director
If the study goal is to understand how management strategies affect system processes and agronomic and environmental outcomes, then the team will want to develop system–treatments that reflect best practices for each management strategy. For example, a study could compare conventional tillage to no-till, organic to conventional, or varying levels of intensification.
If the team wants to understand how diverse, large agricultural systems function relative to managed or unmanaged ecosystems, then the study may need to include agroforestry or woody biomass treatments or unmanaged ecosystems such as old fields in various stages of succession. (See Table 3.1 for examples of projects focusing on this kind of research, including the University of Michigan’s Kellogg Biological Station, the US National Science Foundation’s Long-Term Ecological Research Network, and the Center for Environmental Farming Systems in North Carolina.)
Design Considerations
In simulated agricultural research projects, several aspects of design differ from typical replicated plot experiments. Three issues deserve special attention: plot size, management regimes within treatments, and the use of supplemental, small-scale experiments.
Plot Size
Systems experiments usually require much larger plots than typical experiment station plots, partly to accommodate farm-scale equipment. Using farm-scale equipment offers two main advantages: (1) farmers tend to view results more favorably if the system treatments are managed with the equipment they will be using, and (2) it enables researchers to run accurate energy and economic analyses. Moreover, plot size has important consequences for the experiment itself. Larger plots reduce the significance of edge effects—the influence of differences in microclimate and weed pressure along field margins—and tillage impacts, both of which can become more problematic over time. Large plots ensure that there is plenty of space for sample collection by multiple lab groups, or for experiments to be embedded in the main plots. For example, in long-term experiments, weed pressure can be exacerbated by species that mature and produce seeds along plot edges. In addition, any study that includes research on highly mobile organisms (e.g., arthropods) will require very large plots, and in some cases adequate space is not available. For example, some characteristics of arthropod communities can only be studied using real farm sites because simulated systems cannot be made large enough to mimic on-farm conditions. Likewise, moldboard plowing in small plots can result in poor seed-bed preparation and dead furrows. Excessive wheel traffic can also be a problem in small plots. The team will need to weigh the costs of maintaining a larger experiment against the benefits of larger plots. For example, large plots can be impractical if the study crops are expensive to grow and harvest (such as vegetables that are sequentially hand harvested).
Management Regimes
In traditional factorial experiments, the same crops are grown at the same time. Researchers seek to eliminate crop species as a source of variability so they can study other factors such as tillage or fertility management. In many agricultural systems projects, even those designed as replicated systems comparisons, the treatments being compared most likely will have different crop rotations, and the same crops might not be grown in the same year. During design, remember that crop species is just one of many factors that affect the overall performance of a system. In a systems experiment, the entire system, including the treatments, is the unit of study.
There are two ways to create rotations for each system:
- Accept rotational differences as a factor distinguishing the system treatments. If the goal is to replicate and understand distinct cropping systems, then it might not be necessary to emphasize the performance of specific crops across the systems. Often, these differences in rotation are essential for defining systems that make sense from a farming perspective. For example, a major difference between organic and conventional systems is rotational sequence, because organic systems rely on rotation as part of pest management, whereas conventional systems have access to inputs such as fertilizers and pesticides. If the plan is to grow different crops each year, consider using economic assessments to compare the performance of system treatments (e.g., the cost of production relative to yields and crop value), or consider comparing yields relative to county averages (e.g., “Yields were 10 percent above and 20 percent below county averages in cropping systems 1 and 2, respectively”). The study could also compare soil properties and other attributes to the stage of crop development (e.g., soil health properties can be compared six weeks after planting or at crop senescence). Finally, the results for a particular year become less important as the experiment continues, because trends can be analyzed over time and from multiyear averages of crop-related data. For instance, after 10 years of data collection, a team can examine yield trends over time, so rotational differences across the treatments become less important.
- Design the experiment to accommodate rotational differences and allow for comparison of crops in the same growing season. Rotations can be designed so that the same crop will occur at certain points in the rotation. For example, the team could design three-year and six-year rotations so that corn occurs in the same growing season at least once per rotation cycle. A split-plot design provides the strongest approach to allowing for rotational differences while maximizing opportunities to compare crops because it can accommodate different entry points for each cropping system (Peterson et al., 1999). The number of split plots is determined by the length of the rotation cycle, so that the entire rotation is represented in any given year (Figure 3.4). The use of split plots to represent each entry point in the rotation is an extremely effective design that strengthens the power of the experiment to detect management and/or climate interactions and to directly compare crop performance within management regimes; however, it greatly expands the size and complexity of the experiment. Several cropping systems studies have taken an intermediate approach, using a split-plot design to accommodate more than one point in the rotation each year (usually two to three) without representing the entire rotation every year.
Split Plot. In a split-plot experiment, there are two levels of experimental units: whole plots, and split plots (subplots) within these plots. Split plots allow for an additional variable to be tested within the main experiment. Randomization is used for both the whole plot and the subplots.
Supplemental Satellite Experiments and Nested Subplots
Many agricultural systems experiments include smaller experiments located outside and/or inside the main plots to test new management practices or to solve management problems occurring in the main experiment. Smaller experiments (“satellite trials”) outside of the main plots often consist of small factorial trials intended to solve a problem or test new equipment. These short-term, quick assessments can pretest new varieties, planting densities or techniques for incorporating cover crops. Satellite trials do not account for all variables operating within the larger experiment, but by using them to screen practices, the team can improve management while reducing risks to the main experiment.
Subplots embedded within the larger experiment can be used to test hypotheses and are effective for teasing out underlying mechanistic differences across the systems. When using embedded subplots, only one factor is varied so that specific cause-and-effect relationships within the management system can be investigated. For example, small, paired plots could be used to determine whether weed pressure affects crop yields. Treatments could include a plot in which weeds missed by the normal weed control practices are removed by hand and a “control” plot in which ambient weeds are not removed. (See p. 63 in chapter 4 for a specific discussion of the use of subplots.)
Long-Term Experiments
Many ecosystem processes, particularly those related to soil organic matter dynamics, cannot be adequately studied in less than a decade. To produce useful information, simulated agricultural system studies need to go through two to three rotation cycles, which requires a minimum of 10 to 12 years. Results from the first rotation cycle often represent a transitional phase and do not generally reflect the potential performance of a newly established system.
For long-term studies, establish methods for archiving research protocols, samples and data. Develop a master document to archive field notes and descriptions of weather, field operations and sample collection, along with any observations of unique factors that may have influenced system performance. These may include variables such as weather patterns that interfered with management operations or crop growth. Also document any deviations in management or errors in operations for specific plots. If not adequately catalogued, these events may be lost to future researchers who are trying to make sense of archived data. Also include a system for updating the agricultural practices to keep the experiment relevant (Aref and Wander, 1997). Finally, have a mechanism for maintaining continuity of management regimes, sample collection, analytical techniques and storage methods so that consistency does not depend on a single technician or graduate student. Identify at least one person, usually the team leader, who will commit to keeping the data and archived samples organized and accessible so that the research can continue to build on the early findings of the experiment.